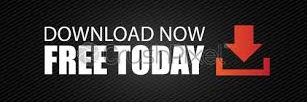
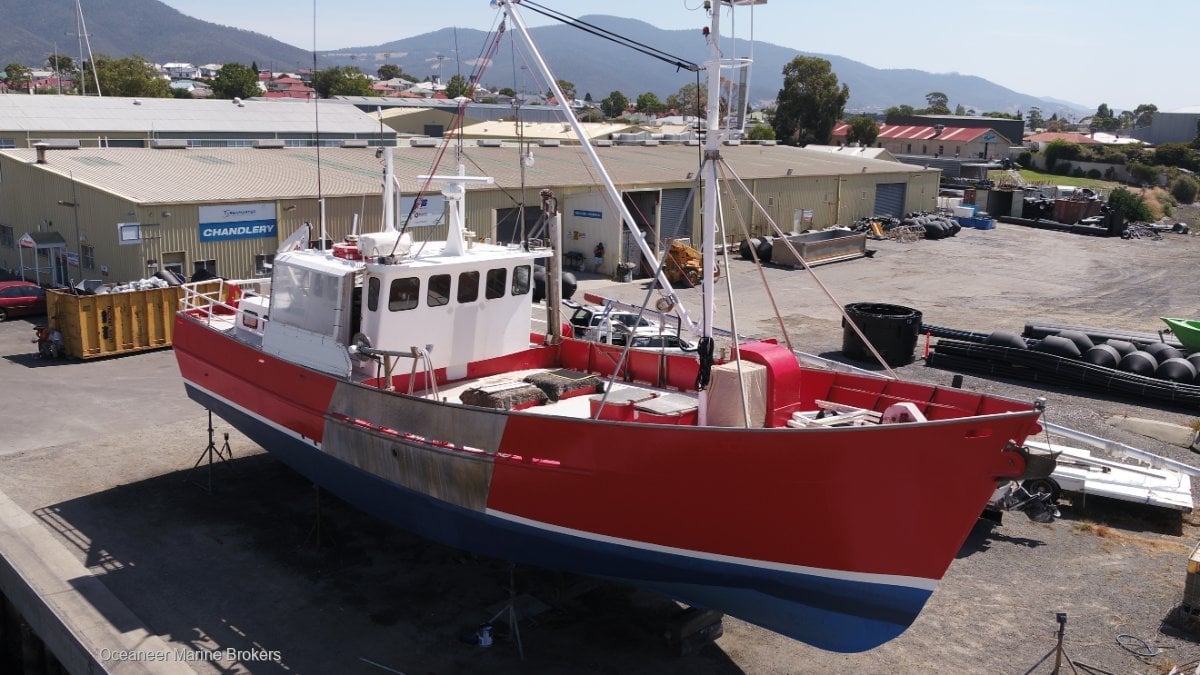
Thus, the package implements a single S3 generic method ( margins()) that can be easily generalized for any type of model implemented in R. This package aims to correctly calculate marginal effects that include complex terms and provide a uniform interface for doing those calculations. Given the challenges of interpreting the contribution of a given regressor in any model that includes quadratic terms, multiplicative interactions, a non-linear transformation, or other complexities, there is a clear need for a simple, consistent way to estimate marginal effects for popular statistical models. interactionTest provides some additional useful functionality for controlling the false discovery rate when making such plots and interpretations, but is again not a general tool for marginal effect estimation. interflex, interplot, and plotMElm provide functionality simply for plotting quantities of interest from multiplicative interaction terms in models but do not appear to support general marginal effects displays (in either tabular or graphical form), while visreg provides a more general plotting function but no tabular output. Unfortunately, none of these packages implement marginal effects correctly (i.e., correctly account for interrelated variables such as interaction terms (e.g., a:b) or power terms (e.g., I(a^2)) and the packages all implement quite different interfaces for different types of models. Among these are car, alr3, mfx, erer, among others. Notably, several packages provide estimates of marginal effects for different types of models.

Other than this relatively new package on the scene, no packages implement appropriate marginal effect estimates. The closest approximation is modmarg, which does one-variable-at-a-time estimation of marginal effects is quite robust. While other Stata modules have provided functionality for deriving quantities of interest from regression estimates (e.g., Clarify), none has done so with the simplicity and genearlity of margins.īy comparison, R has no robust functionality in the base tools for drawing out marginal effects from model estimates (though the S3 predict() methods implement some of the functionality for computing fitted/predicted values). It also represents a significant improvement over Stata’s previous marginal effects command - mfx - which was subject to various well-known bugs. It works with nearly any kind of statistical model and estimation procedure, including OLS, generalized linear models, panel regression models, and so forth. Stata’s margins command is incredibly robust.
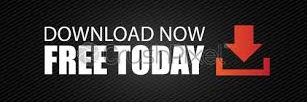